In recent years, split learning (SL) has emerged as a promising distributed learning framework that can utilize big data in parallel without privacy leakage while reducing client-side computing resources. In the initial implementation of SL, however, the server serves multiple clients sequentially incurring high latency. Parallel implementation of SL can alleviate this latency problem, but existing Parallel SL algorithms compromise scalability due to its fundamental structural problem. To this end, our previous works have proposed two scalable Parallel SL algorithms, dubbed SGLR and LocFedMix-SL, by solving the aforementioned fundamental problem of the Parallel SL structure. In this article, we propose a novel Parallel SL framework, coined Mix2SFL, that can ameliorate both accuracy and communication-efficiency while still ensuring scalability. Mix2SFL first supplies more samples to the server through a manifold mixup between the smashed data uploaded to the server as in SmashMix of LocFedMix-SL, and then averages the split-layer gradient as in GradMix of SGLR, followed by local model aggregation as in SFL. Numerical evaluation corroborates that Mix2SFL achieves improved performance in both accuracy and latency compared to the state-of-the-art SL algorithm with scalability
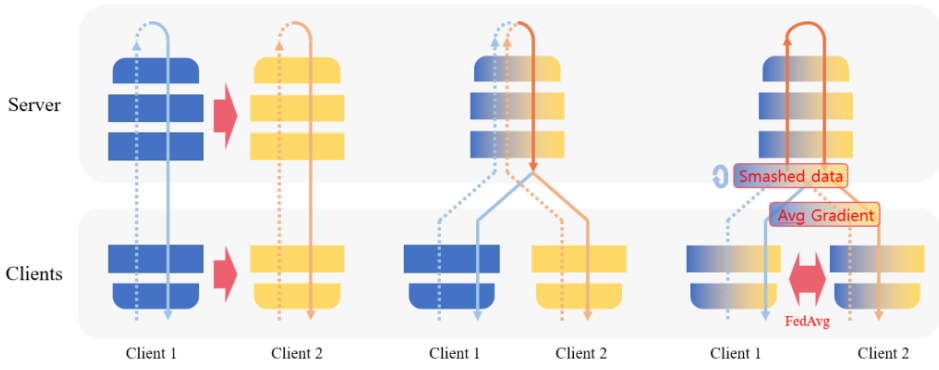
Full Paper: S. Oh, H. Nam, J. Park, P. Vepakomma, R. Raskar, M. Bennis, and S.-L. Kim, ''Mix2SFL: Two-Way Mixup for Scalable, Accurate, and Communication-Efficient Split Federated Learning," accepted to IEEE Transactions on Big Data
留言